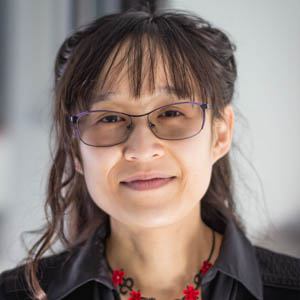
A super-cool paper by Sue Ann Koay, who did this work as a joint postdoc with the Tank lab, is now out in Neuron. The paper contains many beautiful analyses. Among them, Sue Ann shows how efficient coding is implemented at the level of populations.
- Sue Ann Koay, Adam S. Charles, Stephan Y. Thiberge, Carlos D Brody, and David W. Tank, “Sequential and Efficient neural-population coding of complex task information“, Neuron 2021.
Recent work has highlighted that many types of variables are represented in each neocortical area. How can these many neural representations be organized together without interference and coherently maintained/updated through time? We recorded from excitatory neural populations in posterior cortices as mice performed a complex, dynamic task involving multiple interrelated variables. The neural encoding implied that highly correlated task variables were represented by less-correlated neural population modes, while pairs of neurons exhibited a spectrum of signal correlations. This finding relates to principles of efficient coding, but notably utilizes neural population modes as the encoding unit and suggests partial whitening of task-specific information where different variables are represented with different signal-to-noise levels. Remarkably, this encoding function was multiplexed with sequential neural dynamics yet reliably followed changes in task-variable correlations throughout the trial. We suggest that neural circuits can implement time-dependent encodings in a simple way using random sequential dynamics as a temporal scaffold.