HHMI has decided to renew Carlos Brody’s appointment as Investigator until 2032. We get their backing to continue doing the very best science that we can! 💪🏼
► Sep 2024: congratulations to Marino Pagan on his paper being accepted at Nature
The ability to flexibly switch our response to external stimuli according to contextual information is critical for successful interactions with a complex world. Context-dependent computations are necessary across many domains, yet their neural implementations remain poorly understood. Here we developed a novel behavioral task in rats to study context-dependent selection and accumulation of evidence for decision-making. Under assumptions supported by both monkey and rat data, we first show mathematically that a network can solve this problem through a combination of three defined components. These components can be identified and tested directly with experimental data. We further show that existing electrophysiological and modeling data are compatible with the full variety of possible combinations of these components, suggesting that different individuals could use different component combinations. To study variability across individual subjects, we developed automated, high-throughput methods to train rats on our task, and we trained many subjects on it. Consistent with theoretical predictions, neural and behavioral analyses revealed substantial heterogeneity across rats, despite uniformly good task performance. Our theory further predicts a specific link between behavioral and neural signatures, which was robustly supported in the data. In summary, our results provide a new experimentally-supported theoretical framework to analyze individual variability in biological and artificial systems performing flexible decision-making tasks, they open the door to cellular-resolution studies of individual variability in higher cognition, and they provide insights into neural mechanisms of context-dependent computation more generally.
► Nov 2022: new preprint from Marino Pagan on neural mechanisms of flexible decision-making
New preprint [HERE], on work led by postdoc Marino Pagan, together with a great team of collaborators.
For flexible, context-dependent decision-making, the space of network solutions is much larger than previously appreciated.
Moreover, variability across and even within individuals on where they are in this space is the underlying variable that jointly explains neural and behavioral variability.
► Jun 2022: Edward Nieh accepts faculty position at University of Virginia
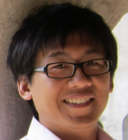
Congratulations to Edward Nieh, who has accepted a faculty position at the University of Virginia, in the Department of Pharmacology, starting May 1, 2023!!! 🎉
► Jun 2022: Diksha Gupta wins Best Paper Award at RLDM conference
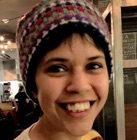
Graduate student Diksha Gupta’s talk, on ”An explanatory link between history biases and lapses” has won the Best Paper Award at the RLDM 2022 Conference! Congratulations Diksha!
► Mar 2022: Brian DePasquale accepts faculty position at Boston University
Congratulations to postdoc Brian DePasquale, who has accepted a faculty position at Boston University, to start in January 2023!!! 🎉
► Jan 2022: Emily Dennis starts a new position as Group Leader at HHMI’s Janelia Research Campus
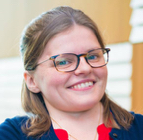
Congratulations to Emily Dennis, who is starting up her own group at Janelia!
► Jan 2022: Sue Ann Koay publishes in Neuron on efficient coding at the neural population level
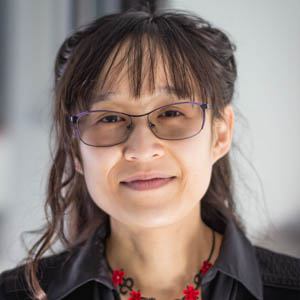
A super-cool paper by Sue Ann Koay, who did this work as a joint postdoc with the Tank lab, is now out in Neuron. The paper contains many beautiful analyses. Among them, Sue Ann shows how efficient coding is implemented at the level of populations.
- Sue Ann Koay, Adam S. Charles, Stephan Y. Thiberge, Carlos D Brody, and David W. Tank, “Sequential and Efficient neural-population coding of complex task information“, Neuron 2021.
Recent work has highlighted that many types of variables are represented in each neocortical area. How can these many neural representations be organized together without interference and coherently maintained/updated through time? We recorded from excitatory neural populations in posterior cortices as mice performed a complex, dynamic task involving multiple interrelated variables. The neural encoding implied that highly correlated task variables were represented by less-correlated neural population modes, while pairs of neurons exhibited a spectrum of signal correlations. This finding relates to principles of efficient coding, but notably utilizes neural population modes as the encoding unit and suggests partial whitening of task-specific information where different variables are represented with different signal-to-noise levels. Remarkably, this encoding function was multiplexed with sequential neural dynamics yet reliably followed changes in task-variable correlations throughout the trial. We suggest that neural circuits can implement time-dependent encodings in a simple way using random sequential dynamics as a temporal scaffold.
►November 2021: Ahmed El Hady takes up a new position as Group Leader in the Department of Collective Behavior at the Max Planck Institute of Animal Behavior
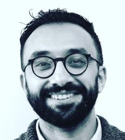
Congratulations to Ahmed el Hady, who is starting his group in the Department of Collective Behavior at the Max Planck Institute for Animal Behavior in Germany!
► Aug 2021: Marino Pagan wins a SFARI Bridge to Independence Award
Congratulations to postdoc Marino Pagan, who is one of six new SFARI Bridge to Independence Fellows! This is a very competitive award. As the SFARI web sites describes, “Launched in 2015, the program is aimed at Ph.D.- and M.D.-holding scientists with an interest in autism research who are currently in training positions but intend to seek tenure-track faculty positions at a U.S. or Canadian research institution during the upcoming academic year. Fellows will receive a commitment of $495,000 over three years, activated upon assumption of their faculty positions.”